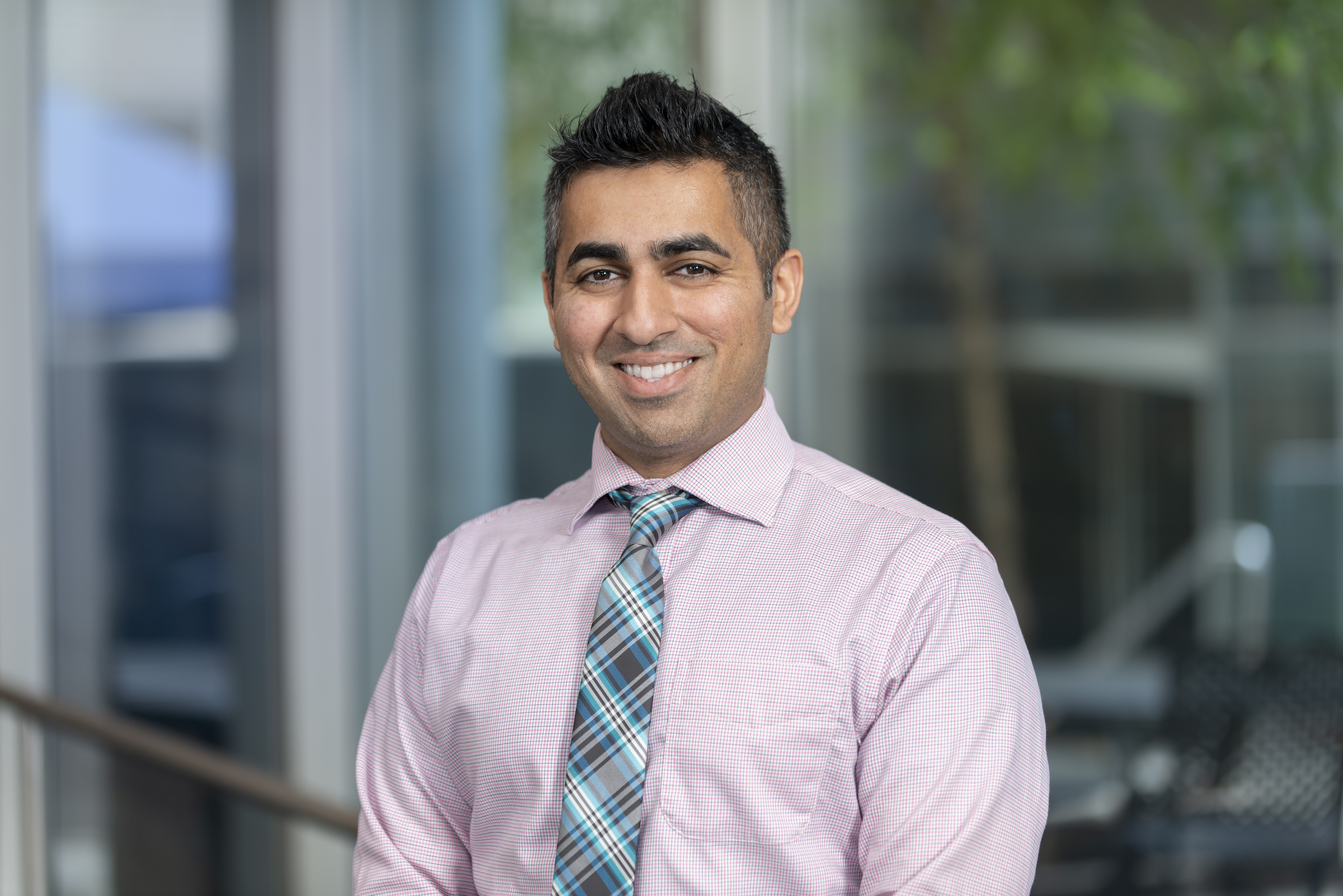
Research
Radiological imaging is a cornerstone of oncological diagnosis and treatment, offering a detailed glimpse into the body's interior. However, the sheer volume and complexity of medical imaging data pose significant challenges to the clinical integration of artificial intelligent (AI) tools for radiological applications. Dr. Mahmood's lab aims to leverage quantitative information derived from medical images that are invisible to the human eye to enhance cancer diagnosis and treatment.
With a strong foundation in medical imaging physics, Dr. Mahmood uses advanced AI techniques to analyze complex imaging datasets, prioritizing data quality and reliability. His current research focuses on methods to validate quantitative imaging features used to characterize the tumor microenvironment objectively and develop large-scale datasets for training machines to classify cancer found on CT scans accurately. With the assistance of a summer student he successfully mentored, he laid the groundwork to develop 3Dprinted phantoms that replicate the complex anatomical shape, micro-texture, and radioopaqueness of tumors detected on CT scans. Two critical use cases of the phantoms are that they provide a real-world benchmark for validating the accuracy of quantitative imaging features, and they enhance AI diagnostic tools' reliability and clinical relevance by offering a physical counterpart to digital images, ensuring algorithmic predictions are rooted in tangible reality. This approach bridges the gap between physical and digital realms and ensures the creation of technically advanced, dependable, and clinically significant AI-driven diagnostics.
An integral part of Dr. Mahmood's research is the ethical deployment of AI in medical imaging. His commitment to addressing AI’s potential biases and risks underscores his dedication to advancing diagnostic accuracy while prioritizing patient safety and equity.
Current projects:
- AI-driven tumor characterization.
- 3D printing for imaging validation.
- Ethical radiological AI tools in oncology.
- QA methodologies for AI tools.
Bio
Usman is a board-certified diagnostic medical physicist with the American Board of Radiology, possessing a diverse background in medical physics that encompasses training and expertise in data science, machine learning, and the quantitative analysis of medical image quality. He earned his B.S. in Physics, graduating cum laude, from Stony Brook University, and subsequently obtained an M.S. in Medical Physics from Columbia University. Beginning his career as a diagnostic medical physicist, Usman furthered his education while working at Memorial Sloan Kettering Cancer Center (MSKCC) by completing a Ph.D. from the Rochester Institute of Technology. His doctoral work focused on developing deep learning systems for radiological applications. Currently, Usman serves as an assistant attending physicist at MSKCC and is the course director for the X-ray methods and computed tomography physics course within Weill Cornell Medical College's biomedical imaging graduate program.
Distinctions:
- 2020 MSK (Memorial Sloan Kettering) Physics Clinical Service Award for work in CT
- 2018 President of Radiological and Medical Physics Association of NY
- 2014 Diplomate, American Board of Radiology - Diagnostic Imaging Physics
Selected Publications:
Mahmood, U., Shukla-Dave, A., Chan, H. P., Drukker, K., Samala, R. K., Chen, Q., ... & Hadjiiski, L. (2024). Artificial intelligence in medicine: mitigating risks and maximizing benefits via quality assurance, quality control, and acceptance testing. BJR| Artificial Intelligence, 1(1), ubae003.
Roemer, G., Li, A., Mahmood, U., Dauer, L., & Bellamy, M. (2024). Artificial intelligence model GPT4 narrowly fails simulated radiological protection exam. Journal of Radiological Protection, 44(1), 013502.
Oh JH, Apte AP, Katsoulakis E, Riaz N, Hatzoglou V, Yu Y, Mahmood U, Veeraraghavan H, Pouryahya M, Iyer A, Shukla-Dave A, Tannenbaum A, Lee NY, Deasy JO. Reproducibility of radiomic features using network analysis and its application in Wasserstein k-means clustering. J Med Imaging (Bellingham). 2021 May;8(3):031904. doi: 10.1117/1.JMI.8.3.031904. Epub 2021 Apr 30.
Mahmood U, Apte A, Kanan C, Bates DDB, Corrias G, Manneli L, Oh JH, Erdi YE, Nguyen J, O'Deasy J, Shukla-Dave A. Quality control of radiomic features using 3D-printed CT phantoms. J Med Imaging (Bellingham). 2021 May;8(3):033505. doi: 10.1117/1.JMI.8.3.033505. Epub 2021 Jun 29.
Mahmood U, Horvat N, Horvat JV, Ryan D, Gao Y, Carollo G, DeOcampo R, Do RK, Katz S, Gerst S, Schmidtlein CR, Dauer L, Erdi Y, Mannelli L. Rapid switching kVp dual energy CT: Value of reconstructed dual energy CT images and organ dose assessment in multiphasic liver CT exams. Eur J Radiol. 2018 May; 102:102-108. doi: 10.1016/j.ejrad.2018.02.022. Epub 2018 Feb 19.