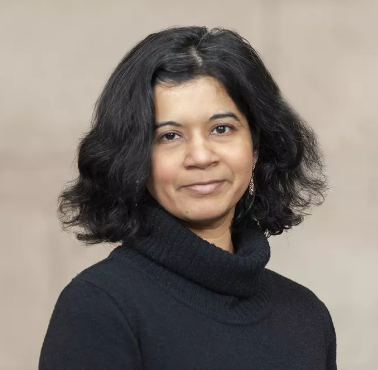
Research
I am interested in developing automated methods to analyze images to extract and combine relevant information from serial imaging and non-imaging modalities to predict cancer treatment outcomes and translate AI models for improving patient treatment outcomes and quality of life.
We combine techniques from computer vision and advanced machine learning to segment cancers, monitor response, and early detect cancer treatment resistance by analyzing longitudinal changes from routinely acquired CT and MRI images. We also develop new deep learning-based deformable image registration methods to help solve challenging problems in radiotherapy including, extracting objective estimates of delivered radiation to organs and tumors during treatment course, synthesize realistic virtual digital twins of patients to predict motion of highly mobile organs to help validate image registration and help towards improving adaptive treatment planning using estimates of cumulative organ and tumor dose, and provide methods to perform population-level outcomes studies. As we develop new AI models and implement them for routine clinical use, we are also studying the bias in such models and creating fair AI models considering patient gender, race, and demographics.
Some key new AI models developed in my lab:
(a) A cross-modality distillation approach to segment lung tumors from low soft tissue contrast cone-beam CT scans
(b) Medical image foundation model called self-supervised masked image transformer (SMIT) that is now also implemented into the MSK radiotherapy clinic for automatically segmenting several organs from brain to pelvis.
(c) New deformable image registration method to model large anatomic deformations occurring at both during (intra-) and between (inter-) radiation treatment fraction that can both segment highly mobile organs in the abdomen and estimate radiation dose delivered to these organs during radiation treatment. This model called ProRSeg is in the final stages of implementation in the MSK radiotherapy clinic for MR guided radiation treatments.
(d) Multiple medical image segmentation methods developed in my lab are now implemented in routine radiation treatment planning applied to multiple disease sites for conventional and magnetic resonance imaging guided radiation treatments at Memorial Sloan Kettering since 2019.
Current Projects:
- Improving inference capability of AI methods
- Longitudinal tumor treatment response monitoring
- Multitasked deep learning for adaptive radiotherapy
- Improving Fairness of AI models
Bio
Harini received a PhD in computer science in 2006 from the University of Minnesota, Twin Cities, where she developed computer vision algorithms combining semi-supervised learning methods for interpreting videos of natural images. She then worked as a postdoctoral fellow in the Computer Science Department at Carnegie Mellon, where she combined computer vision methods with classical AI planning methods into human robot interaction based learning applied to humanoid robots navigating and performing sequences of tasks in indoor environments. Subsequently, she worked as a computer vision scientist for General Electric Research, developing image analysis algorithms for interactive segmentation with active learning applied to cancer segmentation from magnetic resonance and computed tomography scans. At Memorial Sloan Kettering she has been developing and clinically translating AI methods applied to automated segmentation of normal tissues involved as organs at risk for radiation therapy as well as tumors, longitudinal analysis of images to diagnose treatment response, detect early tumor recurrence, and predict treatment outcomes for multiple cancers.
Distinctions:
- Serve as Chair of Joint Working Group Research Seed Funding Initiative at the American Association of Medical Physicists
- Serve as Associate Editor for Medical Physics and International Journal for Radiation Oncology, Biology, Physics
- Four different AI models developed by my lab have now been used in the MSK radiotherapy clinic since June 2019 with more than 15,000 treatment courses performed using our models
- Abstracts from my group honored with “Best in Physics” in the years 2013 and 2018
- Awarded for research excellence in the Medical Physics department in years 2020 and 2022
- Received R01 awards for developing AI methods for improving safety of lung cancers and AI based virtual digital twins for modeling gastrointestinal organ motion for improving treatment of pancreatic cancers
Selected Publications:
Jiang J, Hong J, Tringale K, Reyngold M, Crane C, Tyagi N, Veeraraghavan H, “Progressively refined deep joint registration segmentation (ProRSeg) of gastrointestinal organs at risk: Application to MRI and cone-beam CT”, Medical Physics. 2023. https://pubmed.ncbi.nlm.nih.gov/37265185/
Simeth J, Jiang J, Nosov A, Wibmer A, Zelefsky M, Tyagi N, Veeraraghavan H, “Deep learning-based dominant index lesion segmentation for MR-guided radiation therapy of prostate cancer”, Medical Physics. 2023. https://pubmed.ncbi.nlm.nih.gov/36856092/
Jiang J, Tyagi N, Tringale K, Crane C, Veeraraghavan H, “Self-supervised 3D anatomy segmentation using self-distilled masked image transformer (SMIT)”, Medical Image Computing and Computer Assisted Interventions 2022. https://pubmed.ncbi.nlm.nih.gov/36468915/
Jiang J, Elguindi S, Berry SL, Onochie I, Cervino L, Deasy JO, Veeraraghavan H. “Nested block self-attention multiple resolution residual network for multiorgan segmentation from CT”, Medical Physics, 2022. https://pubmed.ncbi.nlm.nih.gov/35598077/ (This model is used for auto segmentation of head and neck organs in the MSK radiotherapy clinic).
Thompson HM, Kim JK, Jimenez-Rodriguez RM, Garcia-Aguilar J, Veeraraghavan H. “Deep-learning based model for identifying tumor in endoscopic images from patients with locally advanced rectal cancer treated with total neoadjuvant chemotherapy”, Diseases of Colon and Rectum, 2022. https://pubmed.ncbi.nlm.nih.gov/35358109/